The result ensures more accurate, explainable, and transparent outcomes for LLMs and other generative AI applications.
Neo4j has integrated native vector search as part of its core database capabilities. The result enables customers to achieve richer insights from semantic search and generative AI applications, and serve as long-term memory for LLMs, all while reducing hallucinations.
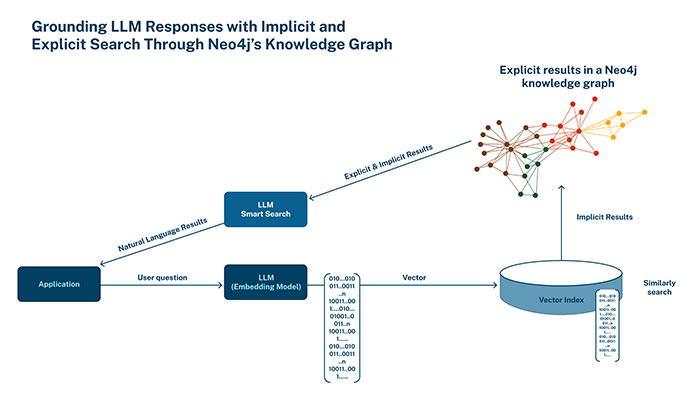
“We see value in combining the implicit relationships uncovered by vectors with the explicit and factual relationships and patterns illuminated by graph.”
Emil Eifrem, Co-Founder and CEO, Neo4j
Neo4j’s graph database can be used to create knowledge graphs, which capture and connect explicit relationships between entities, enabling AI systems to reason, infer, and retrieve relevant information effectively. The result ensures more accurate, explainable, and transparent outcomes for LLMs and other generative AI applications. By contrast, vector searches capture implicit patterns and relationships based on items with similar data characteristics, rather than exact matches, which are useful when searching for similar text or documents, making recommendations, and identifying other patterns.
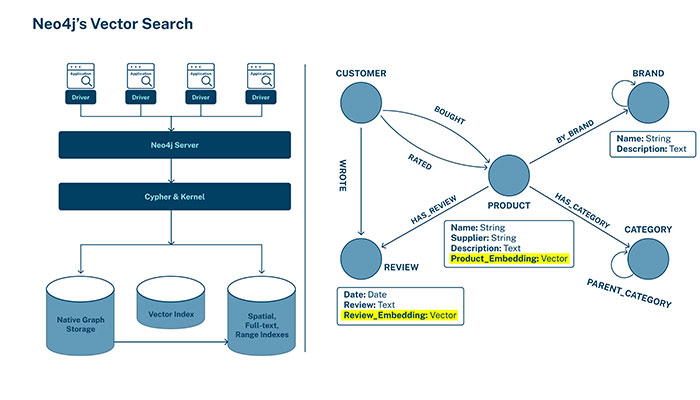
“We see value in combining the implicit relationships uncovered by vectors with the explicit and factual relationships and patterns illuminated by graph,” said Emil Eifrem, Co-Founder and CEO, Neo4j. “Customers when innovating with generative AI also need to trust that the results of their deployments are accurate, transparent, and explainable. With LLMs evolving so dynamically, Neo4j has become foundational for enterprises seeking to push the envelope on what’s possible for their data and their business.”