By: Raffael Marty, Head of X-Labs, Forcepoint
Cracks in Trust and How to Mend Them
Looking at the cybersecurity landscape today, I have to say I’m glad I’m not a CISO. In an ever-evolving world of digital transformation, omni-connected devices and semi-permanent remote workforces, keeping critical data and people safe is a huge challenge. So huge, in fact, that it can’t be done without the implementation of machine learning and automation.
At the core of understanding risk and exposure to an organization, we need to understand its critical data and how that data moves. We can only do so by collecting large quantities of metadata and telemetry about said data and the interactions with it to then apply analytics to make sense and translate it into a risk-based view.
However, developing automated systems is not without its challenges, and in 2021 I believe that machine learning and analytics will fall under tighter scrutiny, as trust in their unbiased nature and fairness, as well as ethical boundaries will be questioned.
Rage at the Machine
We saw headline-grabbing incidents this summer. For example in the United Kingdom, where the government initially decided to let algorithms determine schoolchildren’s exam results. However, the bias which had been baked into this particular algorithm resulted in significant drops in grades: unfairly skewed to lower-income areas, and worse, not taking the teachers’ expertise into account. This resulted in an embarrassing U-turn, where people ended up trumping machines in grading exams.
This is not the first time that algorithms and machine learning systems, trained on biased data sets have been criticized. You will have heard of Microsoft’s Tay chatbot and you may have heard of facial recognition software incorrectly identifying members of the public as criminals. Getting it wrong can have life-changing effects (e.g. for the students or people applying for credit) or could be as “minor” as an inappropriate shopping coupon being sent to a customer.
A number of cybersecurity systems use machine learning to make decisions about whether an action is appropriate (of low risk) for a given user or system. These machine learning systems must be trained on large enough quantities of data and they have to be carefully assessed for bias and accuracy. Get it wrong, apply the controls wrong, and you will experience situations such as a business critical document being incorrectly stopped mid-transit, a sales leader unable to share proposals with a prospect, or other blocks to effective and efficient work. Conversely if the controls are too loose, data can leak out of an organization, causing damaging and costly data breaches.
Finding the Balance in 2021
To build cyber systems that help identify risky users and prevent damaging actions, the data we analyse comes for the most part from looking at a user’s activities. It’s worth saying upfront that user activity monitoring must be done appropriately, and with people’s privacy and the appropriate ethical guidelines in place.
In order to create a virtual picture of users, we can track log on and log off actions. We monitor which files people open, modify, and share. Data is pulled from security systems such as web proxies, network firewalls, endpoint protection and data leak prevention solutions. From this data, risk scores are then computed and the security systems in turn flags inappropriate behaviour and enforces security policies appropriately.
When undertaking this analysis or, in fact, any analysis which uses machine learning or algorithms to make automated decisions which impact people’s lives, we must use a combination of algorithms and human intelligence. Without bringing in human intuition, insights, context and an understanding of psychology, you risk creating algorithms which are themselves biased or make decisions based on flawed or biased data, as discussed above.
In addition to involving human expertise in the algorithms, or in other words, modelling expert knowledge, the right training data and the right data feeding the live analytics is just as important. What constitutes “the right” data? Or, in a similar question, how long is a piece of string! “The right” data is often determined by the problem itself, how the algorithm is constructed, and whether there are reinforcement loops or even explicit expert involvement is possible. The right data means the right amount, the right training set, the right sampling locations, the right trust in the data, the right timeliness, etc. The biggest problem with the ‘right data’ is that it’s almost impossible to define what bias could be present until a false result is observed and it’s potentially too late, harm has been caused.
Using machine learning and algorithms in everyday life is still in its infancy but we see the number of applications grow at stunning pace. In 2021, I expect further applications to fail due to inherent bias, and a lack of expert oversight and control of the algorithms. Not the least problem being that the majority of supervised machine learning algorithms act as a blackbox, making verification either impossible or incredibly hard.
This doesn’t mean that all machine learning algorithms are doomed to failure. The good news is that bias is now being discussed and considered in open groups, alongside the efficacy of algorithms. I hope we will continue to develop explainable algorithms that model expert input. The future of machine learning is bright; the application of algorithms in smart ways is only bounded by our imagination.
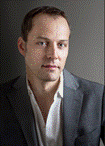
Author: Raffael Marty
Raffael Marty is the Vice President Research and Intelligence at Forcepoint. Raffael heads up X-Labs at Forcepoint, the world’s first dedicated research division that combines deep security expertise with behavioral science research.
X-Labs’ mission is to create the best behavioral-based analytics and security intelligence capability in the cyber-security world, enabling Forcepoint’s human-centric products and services to operate in a predictive fashion.
Previously, Raffael led the Forcepoint Corporate Strategy team. Raffael brings more than 20 years of cybersecurity industry experience across engineering, analytics, research and strategy to Forcepoint. Raffael also provides strategic company growth counsel and guidance and is responsible for how Forcepoint creates value across the Human Point System of products.
Prior to Forcepoint, Marty ran security analytics for Sophos, a leading endpoint and network security company, launched pixlcloud, a visual analytics platform, and Loggly, a cloud-based log management solution. Additionally, Marty held key roles at IBM Research, ArcSight and Splunk and is an expert on established best practices and emerging innovative trends in the big data and security analytics space. Marty is one of the industry’s most respected authorities on security data analytics, big data and visualization. He is the author of “Applied Security Visualization” and is a frequent speaker at global academic and industry events.
Marty holds a master’s degree in computer science from ETH Zurich, Switzerland and is a student of the Japanese tradition of Zen meditation.