Fujitsu Laboratories announced the development of a technology to make AI models more robust against deception attacks. The technology protects against attempts to use forged attack data to trick AI models into making a deliberate misjudgment when AI is used for sequential data consisting of multiple elements.
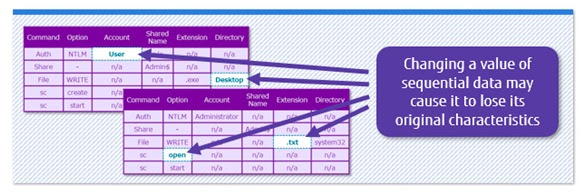
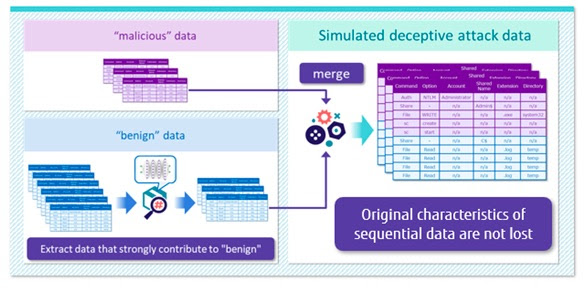
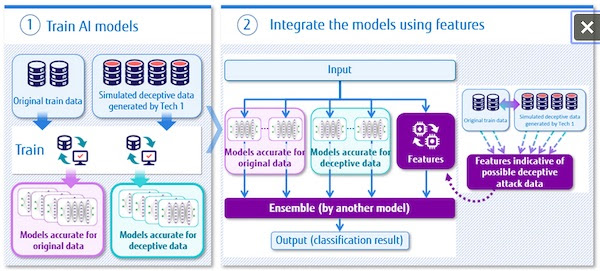
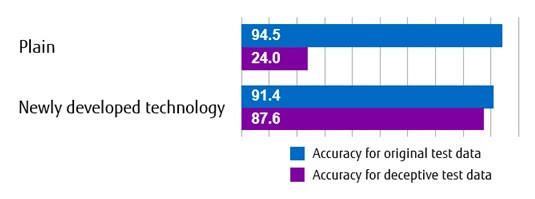
With the use of AI technologies progressing in various fields in recent years, the risk of attacks that intentionally interfere with AI’s ability to make correct judgments represents a source of growing concern. Many suitable conventional security resistance enhancement technologies exist for media data like images and sound. Their application to sequential data such as communication logs and service usage history remains insufficient, however, because of the challenges posed by preparing simulated attack data and the loss of accuracy.
To overcome these challenges, Fujitsu has developed a robustness enhancement technology for AI models applicable to sequential data. This technology automatically generates a large amount of data simulating an attack and combines it with the original training data set to improve resistance to potential deception attacks while maintaining the accuracy of judgment.
By applying this technology to an AI model(1) developed by Fujitsu to judge the necessity of countermeasures against cyber-attacks, it was confirmed that misjudgment of about 88% can be prevented in our own attack test data.
Details of this technology will be announced at the Computer Security Symposium 2020 held from October 26 (Monday) to October 29 (Thursday).
Background
In recent years, AI has been increasingly used to analyze a vast range of data in fields as varied as medicine, social infrastructure, and agriculture. Nevertheless, the existence of security threats peculiar to AI represents a growing threat. Examples include attaching small stickers to road signs to confuse recognition systems and intentionally trying to trick AI models with slightly changed attack data in order to prevent a correct judgment. To help avoid these types of threats, an adversarial training technique has emerged in which simulated attack data created in advance is added to training data so that the AI model is not fooled when it encounters malicious actors.
Previous technologies remain insufficient for dealing with the challenges posed by sequential data, however. AI has a wide range of applications for this type of data, including for the detection of cyber-attacks and credit card fraud, and so a growing need exists to develop technologies that can be applied to sequential data to strengthen resistance against deception attacks.
Issues
One way that cyber-attacks can be detected is through the analysis of communication log data. For instance, when an attacker logs in from the first terminal to another terminal, executes written malware and performs a series of attack operations to spread infection, an AI model can detect the attack from the communication log of such operations. However, attackers disguise attacks by mixing them between legitimate administrative operations, such as collecting server logs or applying patches, which can lead to false negatives in the AI detection model.
In order to apply the adversarial training techniques to such a series of data, it is necessary to automatically generate a large amount of data simulating a deception attack as training data. In the case of media data such as images, it is possible to generate simulated attack data easily without damaging the characteristics of the original data by processing the data in units of pixels that cannot be discriminated by humans. However, in the case of sequential data, it is not clear which element affects the characteristics of the original data, so if you simply process a part of the data, the characteristics of the original data may be lost. (Figure 1) For example, the communication log data used to detect a cyber-attack is a series of log lines consisting of various elements such as the source of communication, the destination of the communication, the account used, the execution command, and the command arguments. In addition, even if simulated attack data can be generated, when it’s used to train AI, it is necessary to be careful not to decrease the judgment accuracy for the original attack data.
Newly Developed Technology
Fujitsu has developed a technology that can automatically generate simulated attack data for training, which can be applied to AI models that analyze sequential data and enable training with less deterioration in the accuracy of attack detection. The features of the developed technology are as follows:
1. Automatic generation of simulated attack data
When creating simulated attack data, we first prepare the original attack data as a base and the data used for impersonation. In the case of cyber-attacks, the attacker wants to disguise malicious operations as benign operations, so the base data is the communication log data of the malicious operation, and the data used for the disguise is the communication log data of the benign operation. Next, the communication log data of benign operations used for the impersonation is analyzed by the AI model before the countermeasure, and the data with the impersonation effect which is easy to be judged as the benign operation is extracted referring to the result. This extracted data is combined with the communication log data of the base malicious operation, and it is generated as simulated attack data. Since the communication log data of the base malicious operation remains unchanged, a large amount of simulated attack data can be generated automatically without losing its original characteristics (Figure 2).
2. Ensemble adversarial training techniques
Using the original learning data set and the simulated attack data set generated with the new technique described above, two kinds of AI models are constructed: an AI model which works accurately for the original learning data and an AI model which works accurately for deception attack data (Figure 3) and the decision results of the two AI models are integrated by ensemble learning(2) using features indicative of possible deception attack data. In the case a cyber-attack is detected, it becomes possible to use ensemble learning to automatically and appropriately train AI models to decide which AI model’s decision should be strongly reflected, since the number of log rows of communication log data and the number of duplicate log rows are used as indicative features. As a result, this technique increases the robustness to deception attacks while keeping loss of accuracy in the original data under control.
Effect
By using the newly developed technology, it is possible to strengthen the resistance of the AI model against deception attacks against sequential data. To demonstrate its effectiveness, Fujitsu applied the newly developed techniques to an AI model that determines the necessity of responding to cyber-attacks. As a result, it was confirmed that the accuracy of judgment against simulated attack test data could be improved to about 88% without lowering the accuracy of judgment against the original test data. In addition, when analyzing the test data of the deception attack that went undetected, it was discovered that it was possible to deal with a simple rule such as judging a combination of specific operations as a deception attack, which made it possible to prevent virtually all deception attacks.
Future Plans
In the future, Fujitsu plans to expand the application of this technology not only to counter cyber-attacks, but also to a variety of other areas to improve the security of systems using AI. In fiscal 2021, Fujitsu additionally aims to commercialize these techniques as part of a security enhancement technology offering.
(1) AI model AI technology that automatically determines whether action needs to be taken in response to a cyberattack. https://bit.ly/31QacSk
(2) Ensemble learning Refers to techniques that improve accuracy by combining results from multiple AI models
About Fujitsu
Fujitsu is the leading Japanese information and communication technology (ICT) company offering a full range of technology products, solutions and services. Approximately 130,000 Fujitsu people support customers in more than 100 countries. We use our experience and the power of ICT to shape the future of society with our customers. Fujitsu Limited (TSE:6702) reported consolidated revenues of 3.9 trillion yen (US$35 billion) for the fiscal year ended March 31, 2020. For more information, please seewww.fujitsu.com.